We know many of you have to become armchair (or armrest – Snowjobs listeners know) meteorologists, too. How accurate are the different weather forecasting models? How different should I expect different weather forecasting models to be? We’ll break down the pros and cons from the perspective of the snow removal professional; each model may be better suited for different uses.
But first – how exactly is a forecast model developed? The easiest way to think about it is as a computer program that takes in current and historical weather observations and uses math and physics formulas to estimate how those conditions will change in the future. These models combine atmospheric information like pressure, wind, and temperature from airport and road weather information (RWIS) stations, Doppler radar, weather balloons, satellites, and other ancillary data points, to diagnose what is happening and predict what is going to happen..
Because the models are driven primarily by current conditions from weather sensors, they typically get less accurate as the forecast goes out in time. Models also tend to be more accurate over terrain that is not as challenging, like the plains, as opposed to mountainous areas where model resolution is too coarse to resolve microclimates.
In the information below, spatial resolution is the length of the bounds of a grid cell (e.g. 3 km resolution = 3 km x 3 km grid cell). Estimates are made for many different variables within that grid cell and also from neighboring grid cells in order to complete a prediction. The model is a grid of these cells that holds onto predictions over consistent time steps. The length of these time steps is the model’s temporal resolution (e.g. hourly timesteps, 1-hr resolution).
RAP or RR
- Stands for: Rapid Refresh
- Origin: NOAA (National Oceanic and Atmospheric Association)
- Pros:
- Updated hourly
- Good for tracking rapidly changing weather
- Cons:
- 13 km resolution
HRRR
- Stands for: High Resolution Rapid Refresh
- Origin: NOAA (National Oceanic and Atmospheric Association)
- Pros:
- Focused specifically on the US, Canada, and Mexico
- Radar data is updated every 15 minutes over 1 hour periods
- 3 km resolution
- More distinct cloud bands (like lake effect and snow squalls)
- Cons:
- Only forecasts out 1.5 days or 36 hours
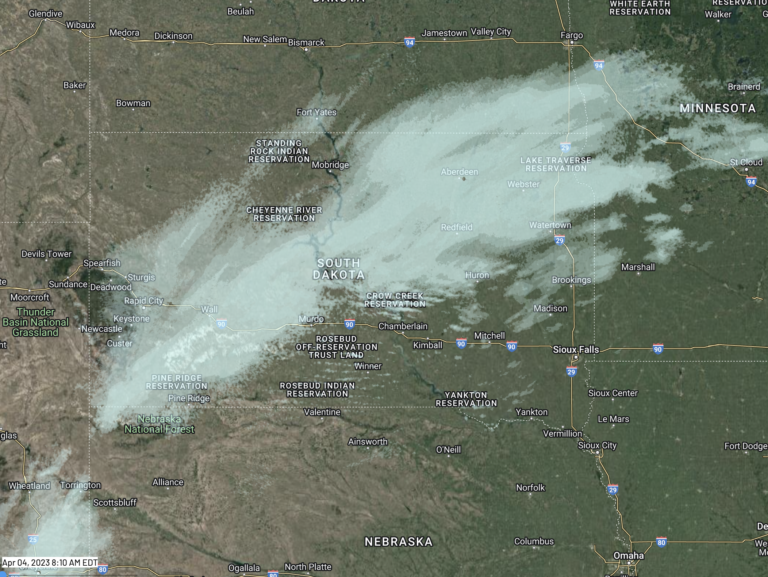
NAM
- Stands for: North American Mesoscale
- Origin: National Centers for Environmental Prediction (NCEP)
- Pros:
- Focused specifically on the US, Canada, and Mexico
- 12 km and 3 km resolutions available
- Cons:
- Only updates twice a day
- Forecasts out 3.5 days or 84 hours
GFS
- Stands for: Global Forecast System
- Origin: National Centers for Environmental Prediction (NCEP)
- Pros:
- Can forecast out to 16 days or 384 hours
- Cons:
- Global model with larger grids of 13km; best for tracking huge storms like hurricanes, not regional or short term events
- Updated every 6 hours
ECMWF
- Stands for: European Centre for Medium-Range Weather Forecasts
- Origin: 18 European countries formed the ECMWF convention in 1975 as an intergovernmental organization to pool Europe’s meteorological expertise, with the objective of improving mid-range forecasts
- Pros:
- Forecasts out to 10 days or 240 hours
- Historically has shown the highest accuracy ratings
- Cons:
- 9 km resolution
- Only updates twice a day
GEM RDPS and GDPS
- Stands for: Global Environmental Multiscale Regional Deterministic Prediction System and Global Deterministic Prediction System
- Origin: Environment Canada
- Pros:
- Variable resolution, enabling large scale monitoring or a “zoomed in” view of a forecast
- Forecasts out to 10 days, updated every hour
- RDPS offers 2.5 km resolution over Canada
- Cons:
- Regional model goes to 10 km resolution for the rest of North America
- Global model uses 25 km resolution and is updated every 12 hours, making it less relevant for those outside Canada
So what does the future of forecasting models look like? The groups above will inevitably continue to refine their models as we add more datapoints (like RWIS devices and other weather observation stations) and get more powerful computers. The more powerful our computers, the more datapoints can be ingested and processed to build a better model. Like most industries, the rise of artificial intelligence will likely heavily influence forecasting in the coming years. From The Weather Company being acquired by IBM to ChatGPT learning to forecast the weather, change is coming, and it’s probably for the benefit of everyone in the industry.
If you are interested in seeing the visual differences between forecast models’ radars, Weather.us has a good resource for this. Sources used in this article include: